Cosine similarity of all written/oral States of the Union is 0.55. A highly ambiguous result, but one that suggests there are likely some differences overlooked by Rule et al. (2015). A change in medium should affect genre features, if only at the margins. The most obvious change is to length, which I pointed out in the last post.
But how to discover lexical differences? One method is naive Bayes classification. Although the method has been described for humanists in a dozen places at this point, I’ll throw my own description into the mix for posterity’s sake.
Naïve Bayes classification occurs in three steps. First, the researcher defines a number of features found in all texts within the corpus, typically a list of the most frequent words. Second, the researcher “shows” the classifier a limited number of texts from the corpus that are labeled according to text type (the training set). Finally, the researcher runs the classifier algorithm on a larger number of texts whose labels are hidden (the test set). Using feature information discovered in the training set, including information about the number of different text types, the classifier attempts to categorize the unknown texts. Another algorithm can then check the classifier’s accuracy rate and return a list of tokens—words, symbols, punctuation—that were most informative in helping the classifier categorize the unknown texts.
More intuitively, the method can be explained with the following example taken from Natural Language Processing with Python. Imagine we have a corpus containing sports texts, automotive texts, and murder mysteries. Figure 2 provides an abstract illustration of the procedure used by the naïve Bayes classifier to categorize the texts according to their features. Loper et al. explain:
In the training corpus, most documents are automotive, so the classifier starts out at a point closer to the “automotive” label. But it then considers the effect of each feature. In this example, the input document contains the word “dark,” which is a weak indicator for murder mysteries, but it also contains the word “football,” which is a strong indicator for sports documents. After every feature has made its contribution, the classifier checks which label it is closest to, and assigns that label to the input.
Each feature influences the classifier; therefore, the number and type of features utilized are important considerations when training a classifier.
Given the SotU corpus’s word count—approximately 2 million words—I decided to use as features the 2,000 most frequent words in the corpus (the top 10%). I ran NLTK’s classifier ten times, randomly shuffling the corpus each time, so the classifier could utilize a new training and test set on each run. The classifier’s average accuracy rate for the ten runs was 86.9%.
After each test run, the classifier returned a list of most informative features, the majority of which were content words, such as ‘authority’ or ‘terrorism’.
However, a problem . . . a direct comparison of these words is not optimal given my goals. I could point out, for example, that ‘authority’ is twice as likely to occur in written than in oral States of the Union; I could also point out that the root ‘terror’ is found almost exclusively in the oral corpus. Nevertheless, these results are unusable for analyzing the effects of media on content. For historical reasons, categorizing the SotU into oral and written addresses is synonymous with coding the texts by century. The vast majority of written addresses were delivered in the nineteenth century; the majority of oral speeches were delivered in the twentieth and twenty-first centuries. Analyzing lexical differences thus runs the risk of uncovering, not variation between oral and written States of the Union (a function of media) but variation between nineteenth and twentieth century usage (a function of changing style preferences) or differences between political events in each century (a function of history). The word ‘authority’ has likely just gone out of style in political speechmaking; ‘terror’ is a function of twenty-first century foreign affairs. There is nothing about medium that influences the use or neglect of these terms. A lexical comparison of written and oral States of the Union must therefore be reduced to features least likely to have been influenced by historical exigency or shifting usage.
In the lists of informative features returned by the naïve Bayes classifier, pronouns and contraction emerged as two features fitting that requirement.
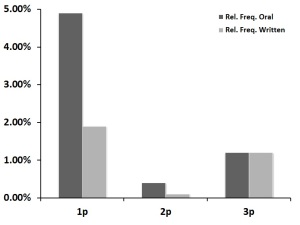
Relative frequencies of first, second, and third person pronouns
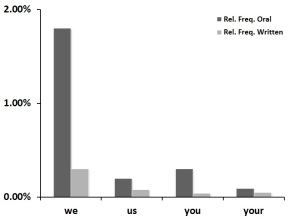
Relative frequencies of select first and second person pronouns
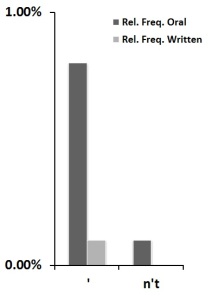
Relative frequencies of apostrophes and negative contraction
It turns out that pronoun usage is a noticeable locus of difference between written and oral States of the Union. The figures above show relative frequencies of first, second, and third person pronouns in the two text categories (the tallies in the first graph contain all pronomial inflections, including reflexives).
As discovered by the naïve Bayes classifier, first and second person pronouns are much more likely to be found in oral speeches than in written addresses. The second graph above displays particularly disparate pronouns: ‘we’, ‘us’, ‘you’, and to a lesser extent, ‘your’. Third person pronouns, however, surface equally in both delivery mediums.
The third graph shows relative frequency rates of apostrophes in general and negative contractions in particular in the two SotU categories. Contraction is another mark of the oral medium. In contrast, written States of the Union display very little contraction; indeed, the relative frequency of negative contraction in the written SotU corpus is functionally zero (only 3 instances). This stark contrast is not a function of changing usage. Negative contraction is attested as far back as the sixteenth century and was well accepted during the nineteenth century; contraction generally is also well attested in nineteenth century texts (see this post at Language Log). However, both today and in the nineteenth century, prescriptive standards dictate that contractions are to be avoided in formal writing, a norm which Sairio (2010) has traced to Swift and Addison in the early 1700s. Thus, if not the written medium directly, then the cultural standards for the written medium have motivated presidents to avoid contraction when working in that medium. Presidents ignore this arbitrary standard as soon as they find themselves speaking before the public.
The conclusion to be drawn from these results should have been obvious from the beginning. The differences between oral and written States of the Union are pretty clearly a function of a president’s willingness or unwillingness to break the wall between himself and his audience. That wall is frequently broken in oral speeches to the public but rarely broken in written addresses to Congress.
As seen above, plural reference (‘we’, ‘us’) and direct audience address (‘you’, ‘your’) are favored rhetorical devices in oral States of the Union but less used in the written documents. The importance underlying this difference is that both features—plural reference and direct audience address—are deliberate disruptions of the ceremonial distance that exists between president and audience during a formal address. This disruption, in my view, can be observed most explicitly in the use of the pronouns ‘we’ and ‘us’. The oral medium motivates presidents to construct, with the use of these first person plurals, an intimate identification between themselves and their audience. Plurality, a professed American value, is encoded grammatically with the use of plural pronouns: president and audience are different and many but are referenced in oral speeches as one unit existing in the same subjective space. Also facilitating a decrease in ceremonial distance, as seen above, is the use of second person ‘you’ at much higher rates in oral than in written States of the Union. I would suggest that the oral medium motivates presidents to call direct attention to the audience and its role in shaping the state of the nation. In other cases, second person pronouns may represent an invitation to the audience to share in the president’s experiences.
Contraction is a secondary feature of the oral medium’s attempt at audience identification. If a president’s goal is to build identification with American citizens and to shorten the ceremonial distance between himself and them, then clearly, no president will adopt a formal diction that eschews contraction. Contraction—either negative or subject-verb—is the informality marker par excellence. Non-contraction, on the other hand, though it may sound “normal” in writing, sounds stilted and excessively proper in speech; the amusing effect of this style of diction can be witnessed in the film True Grit. In a nation comprised of working and middle class individuals, this excessively proper diction would work against the goals of shortening ceremonial distance and constructing identification. Many scholars have noted Ronald Reagan’s use of contraction to affect a “conversational” tone in his States of the Union, but contraction appears as an informality marker across multiple oral speeches in the SotU corpus. In contrast, when a president’s address takes the form of a written document, maintaining ceremonial distance seems to be the general tactic, as presidents follow correct written standards and avoid contractions. The president does not go out of his way to construct identification with his audience (Congress) through informal diction. Instead, the goal of the written medium is to report the details of the state of the nation in a professional, distant manner.
What I think these results indicate is that the State of the Union’s primary audience changes from medium to medium. This fact is signaled even by the salutations in the SotU corpus. The majority of oral addresses delivered via radio or television are explicitly addressed to ‘fellow citizens’ or some other term denoting the American public. In written addresses to Congress, however, the salutation is almost always limited to members of the House and the Senate.
Two lexical effects of this shift in audience are pronoun choice and the use or avoidance of contraction. ‘We’, ‘us’, ‘you’—the frequency of these pronouns drops by fifty percent or more when presidents move from the oral to the written medium, from an address to the public to an address to Congress. The same can be said for contraction. Presidents, it seems, feel less need to construct identification through these informality markers, through plural and second person reference, when their audience is Congress alone. In contrast, audience identification becomes an exigent goal when the citizenry takes part in the State of the Union address.
To put the argument another way, the SotU’s change in medium has historically occurred alongside a change in genre participants. These intimately linked changes motivate different rhetorical choices. Does a president choose or not choose to construct a plural identification between himself and his audience (‘we’,’us’) or to call attention to the audience’s role (‘you’) in shaping the state of the nation? Does a president choose or not choose to use obvious informality markers (i.e., contraction)? The answer depends on medium and on the participants reached via that medium—Congress or the American people.
~~~
Tomorrow, I’ll post results from two 30-run topic models of the written/oral SotU corpora.